ASHVIN WP2 “Design for Productivity and Safety” develops possibilities to allow designers and engineers to account for the aspects of productivity, resource efficiency, and safety early in the design phase. It also experiments with possibilities to establish evidence-based design practices based on historical digital twin data. Deliverable 2.2 entitled “Evaluation and Recommendations for Evidence-Based Design for Productivity, Resource Efficiency, and Safety based on Historical Digital Twin Data” was published in September 2022 along with 11 other major technical deliverables defining the ASHVIN system. This report is related to the task 2.2 “Evidence based design assistant” led by SBP.
The early design phases of construction projects have a major impact on the success of the projects in terms of cost, construction time, global warming potential and other aspects. However, detailed information on the ongoing design is often lacking in these early project phases. This gap can be filled by using information and data of existing infrastructure. The historic data from past projects is collected, processed, analyzed, and evaluated systematically so that it is made into ready input for design. This is the evidence-based design (EBD) assistant’s approach to support design at the early project stages. A database of past footbridge designs provided by the engineering design firm schlaich bergermann partner (sbp) is used as the knowledge database to investigate and validate the potential of using historical data for evidence-based design to improve the design of future projects. Data collection was done manually involving digitising past project data which will be stored in the digital twin for construction. The collected data was cleaned, filtered, and clustered using data analytics methods. Data processing, analyses and evaluation was done using machine learning approaches. The study found that (i) historic data/information is valuable input for design, (ii) historic data/information provides good predictions for performance indicator values (PI-values), and (iii) machine learning models can be used to evaluate and compare accuracies of PI-values. The very limited size of the database and scanty data was a major challenge in the implementation of the approach. However, we recommend that organisations consider growing such knowledge databases to enrich evidence-based design.
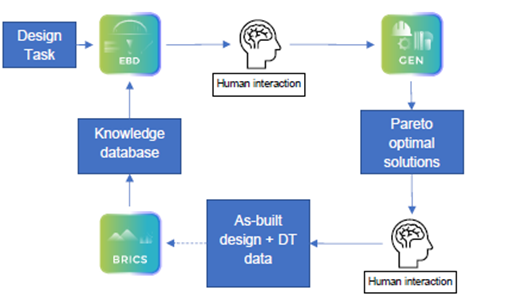
Download the entire report in Open Access via Zenodo.
For more information, visit our Digital Toolkit Page, and connect with us through our LinkedIn or Twitter communities!