During the last project year, the ASHVIN project’s aim was to open the ASHVIN Digital Twin solution to more construction and maintenance projects by collecting their data with a wide range of IoT and image technologies and feeding the collected data to the ASHVIN Digital Twin platform. This goal was set at the beginning of the project to open the project’s innovation and research results for a broad range of stakeholders. Also, we ensure that ASHVIN’s research benefits an important number of building sites and infrastructure assets in Europe beyond the 10 demonstration sites that already contribute to developing and testing the system.
The ASHVIN project, supported by the European Union, gathers 15 European research and industry partners to develop a novel digital twin solution for the building sector. ASHVIN’s Digital Twin solution comprises a set of 10 smart building applications supporting the design, construction and maintenance of buildings and infrastructure assets. These tools are integrated into an IoT platform running back-end and connected to sensors harvesting data from the sites, as well as a game-engine-based Digital Twin platform enabling the visualisation of the collected data.
Now, we present insights from our demo sites dedicated to improving structural and operational safety, thanks to using ASHVIN’s digital twin technologies.
Urban Excavations in Germany, Taiwan and the USA
The approach adopted in T4.4 Data-Driven Risk Management was performed on publicly available data from three urban excavations in Germany, Taiwan and the USA. An approach was taken incorporating geotechnical site investigation data into a numerical excavation model, following the same approach developed for Demonstration Case #10 in ASHVIN. The model is then validated based on the observed measurement data.
To improve future digital twin platforms’ updating efficiency and speed, a machine learning-based “surrogate model” was trained on simulations run in the validated numerical model. This surrogate model approximates the numerical model’s behaviour, understanding the dependencies between the inputs and a specific output. The result is a substantial improvement in computational time (from two minutes per simulation to less than a second) whilst maintaining sufficient accuracy.
These extra demo cases have helped validate the approach developed for Demonstration Case #10, and innovative machine learning algorithms have shown potential pathways for developing quicker and more reliable digital twins that can be updated based on measurement observations during construction.
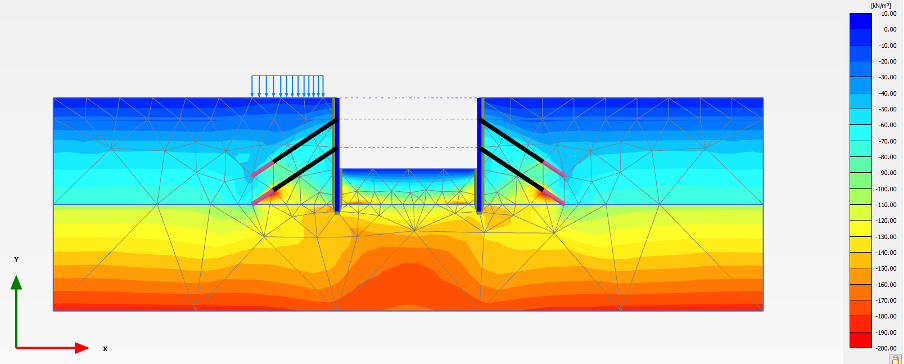
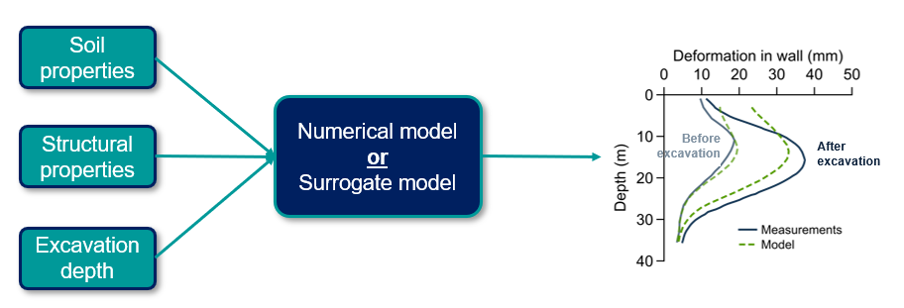
Three Croatian airports to support their maintenance
Three new external demo sites focused on improving the maintenance process of infrastructure assets and increasing structural and operational safety with support from the deployed digital twin technologies. These three airports were located in Zagreb, Pula, and Mali Losinj in Croatia, and they were managed by Infraplan Consulting, the ASHVIN partner who also managed the research and pilots at demo site #3, “Airport runway in Croatia.”
Automated technologies improve the Safety Management Systems at the airports
Three additional airports adopted the methodologies developed in T5.3 and T5.4. They have been inspected by remote sensing technologies, namely unmanned aerial vehicles (UAVs) with different sensors. Since airports are one of the vital transport infrastructure, with a number of complex operations, one of the main objectives for the airport manager is to keep the runway safe and open.
Figure 1: Usage of UAV for runway inspection
The airport maintenance process consists of different procedures and inspections to ensure adequate aircraft operations safety. These inspections include operational surfaces on the airport airside, and the process is performed manually with traditional technologies (with vehicles).
The new approach is to use automated technologies in order to improve efficiency and reduce operational costs and greenhouse gas emissions. An unmanned aerial vehicle represents a potential alternative technology to replace traditional processes. Because of high safety standards, runways are particularly exposed to considerable loading and require daily inspections and monitoring.
The inspector should look for objects, contaminants, and anomalies on the runway surface that may endanger the safe landings and take-offs of aircraft, such as:
- foreign object debris (FOD);
- contaminants on the runway surface such as water, snow, spilled oil or fuel, or rubber deposit etc.;
- cracks and potholes on the runway surface;
- birds and wildlife on or in the vicinity of the runway. Instead of using vehi-cles for all operations, the intention is to replace some of them with UAVs.
Such an approach may bring significant benefits, including reducing air pollution (in line with European Green Deal policy), improving infrastructure inspection efficiency, and increasing anomaly detection accuracy on operational surfaces. Also, this method allows multiple measurements (inspection) per drone operation. Overall, the automated technologies improve the Safety Management System at the airport.
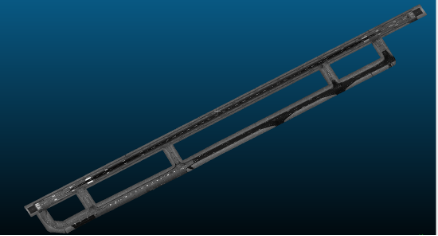
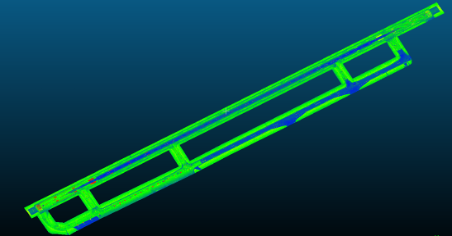
Figure 2 Zagreb airport orthophoto map produced from high resolution images captured by drone (left) and LiDAR scan (right)
Remote sensing technologies improve monitoring processes
For the purpose of digitalisation, inspection and monitoring of runway condition unmanned aerial vehicles (UAVs) were used, equipped with a high-resolution camera, thermal camera and LiDAR scanner. The aim was to replicate and validate the methodology developed for Demo#3 Zadar Airport.
Implementing remote sensing technologies can significantly improve and automate inspection and monitoring processes, enabling the detection of foreign objects or defects at the very early stage (which often can be missed by the human eye), and continuous monitoring of any ongoing deterioration process or damage. The time needed for an inspection will decrease while the quality of inspections will increase.
The collected data is used for the development of BIM or digital twin models, which enable continuous and accurate monitoring of the whole infrastructure, finally being used for the GIS-based (GIS tool from the ASHVIN toolkit) asset management models and optimisation of maintenance planning.
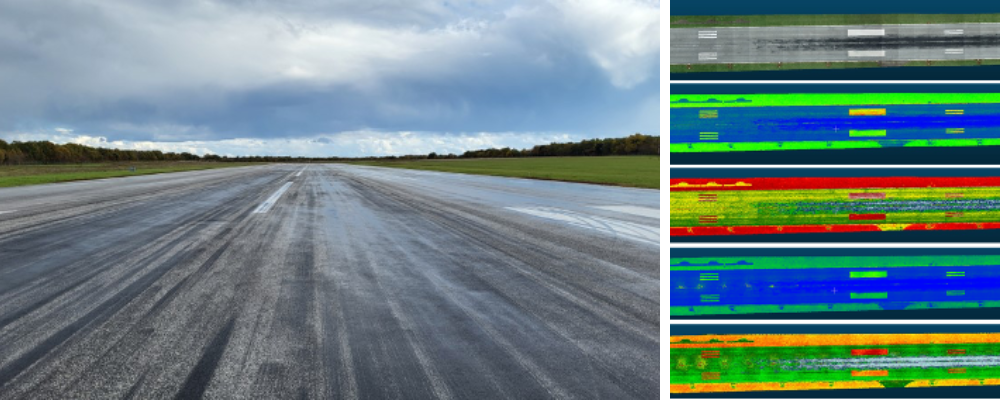
Figure 3 Airport Pula drone flight image (left) and different visualization of the captured runway surface presenting wet/dry conditions of the runway (right)