The ASHVIN project develops an IoT-driven Digital Twin Platform and a digital toolkit supporting the European construction industry to become more productive and safer for workers. The ASHVIN solution can be used in the three construction phases: design, construction and maintenance of building sites.
Early-design Phase Fed with Data from Past Projects
The early design phases of construction projects significantly impact the projects’ success in terms of cost, construction time, global warming potential and other aspects. However, detailed information on the ongoing design is needed, but this data is generally lacking. This gap can be filled by using information and data from existing infrastructure. The ASHVIN team collected historical data from past projects and then processed, analyzed, and evaluated it systematically to make it into ready input for design. This is the evidence-based design (EBD) assistant’s approach to support design at the early project stages. TUB (↗️) leads this part of the ASHVIN research.
Generative design is already successfully used as a design concept in many industries. The basic idea is to use a computer to generate many design alternatives for a given design task and then evaluate them on a set of target variables. In civil engineering, specifically in structural design, this design process has now also found its way into several projects.
The EBD Approach aims towards an audience in the early stage of footbridge design. The audience includes engineers, designers, and decision-makers in public and private institutions.
In the ASHVIN project, a database of past footbridge designs provided by the engineering design firm schlaich bergermann partner (sbp) (↗️) is used. This serves as the knowledge database to investigate and validate the potential of using historic digital twin data for evidence-based design to improve the design of future projects.
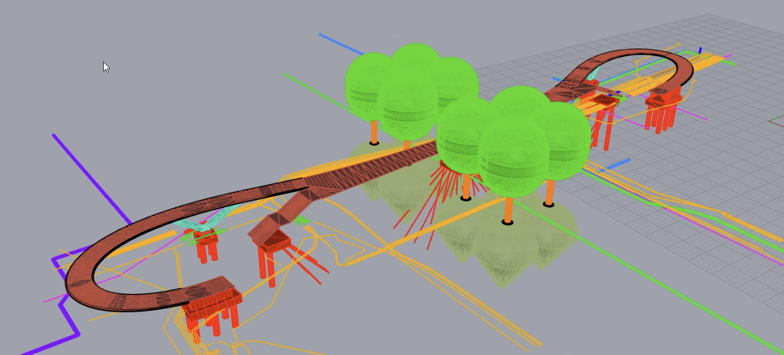
A Footbridge model from Germany, sbp 2022.
Data collection was done manually, involving digitizing past project data stored in the digital twin for construction. The collected data was cleaned, filtered, and clustered using data analytics methods. Data processing, analyses and evaluation were done using machine learning approaches.
Prototypes of Three Smart Building Tools
The study found that historic data/information is valuable input for design, but also, historic data/information provides good predictions for performance indicator values (PI-values), and machine learning models can be used to evaluate and compare the accuracies of PI-values.
Hence, the evidence-based and generative designs are capsulated into three tool prototypes, BRICS, GEN and EBD, part of the ASHVIN toolkit (↗️).
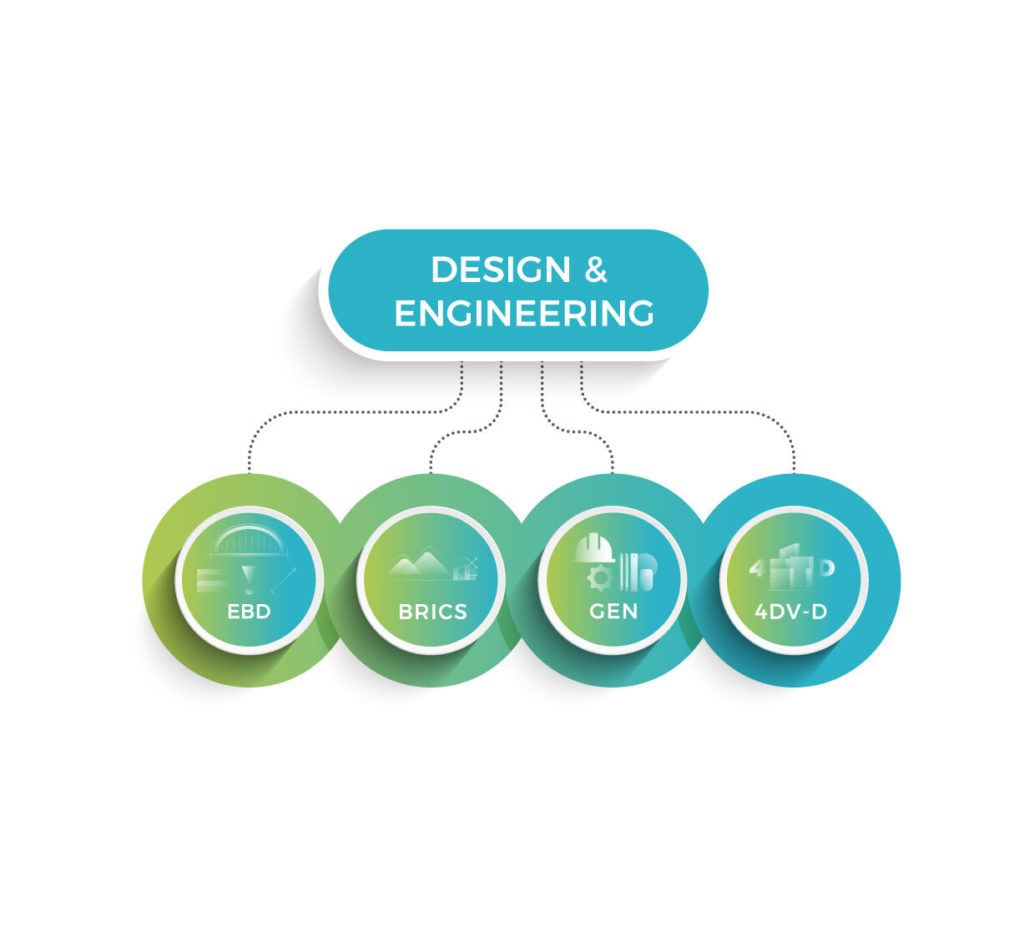
BRICS refers to the Performance Indicator (PI) assessment of solutions in the design space. GEN is the Generative design modeller to support design for productivity, resource efficiency and safety. EBD is the Evidence-Based design support tool for recycling historic data as input at the early project stages
These tools are under demonstration and validation at the ASHVIN demonstration site #8 Footbridge in Germany (↗️.
Towards a Standardised Way of Collecting Data
For the ASHVIN team, the minimal size of the database and scanty data was significant challenge in implementing the approach. However, we recommend that organizations consider growing such knowledge databases to enrich the evidence-based design. The overall process shows the approach’s applicability even though the available database has significant flaws. This motivates the introduction of a digital twin as a standardized way of collecting data on future footbridge design and construction projects.
To learn more about the Evidence Design tool, check out the EDB, GEN and BRICS tutorial videos (↗️).
Also, download Deliverable 2.2 (↗️) “Evaluation and recommendations for evidence-based design for productivity, resource efficiency, and safety based on historical digital twin data.”
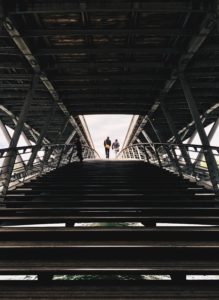
Stay tuned for more ASHVIN results, and connect with us through our LinkedIn or Twitter communities!